Using ML to green HPC and cloud computing
A single cloud computing data center can consume as much power as 50,000 homes. Redpoint AI partnered with a cloud provider to help them conserve energy and reduce costs.
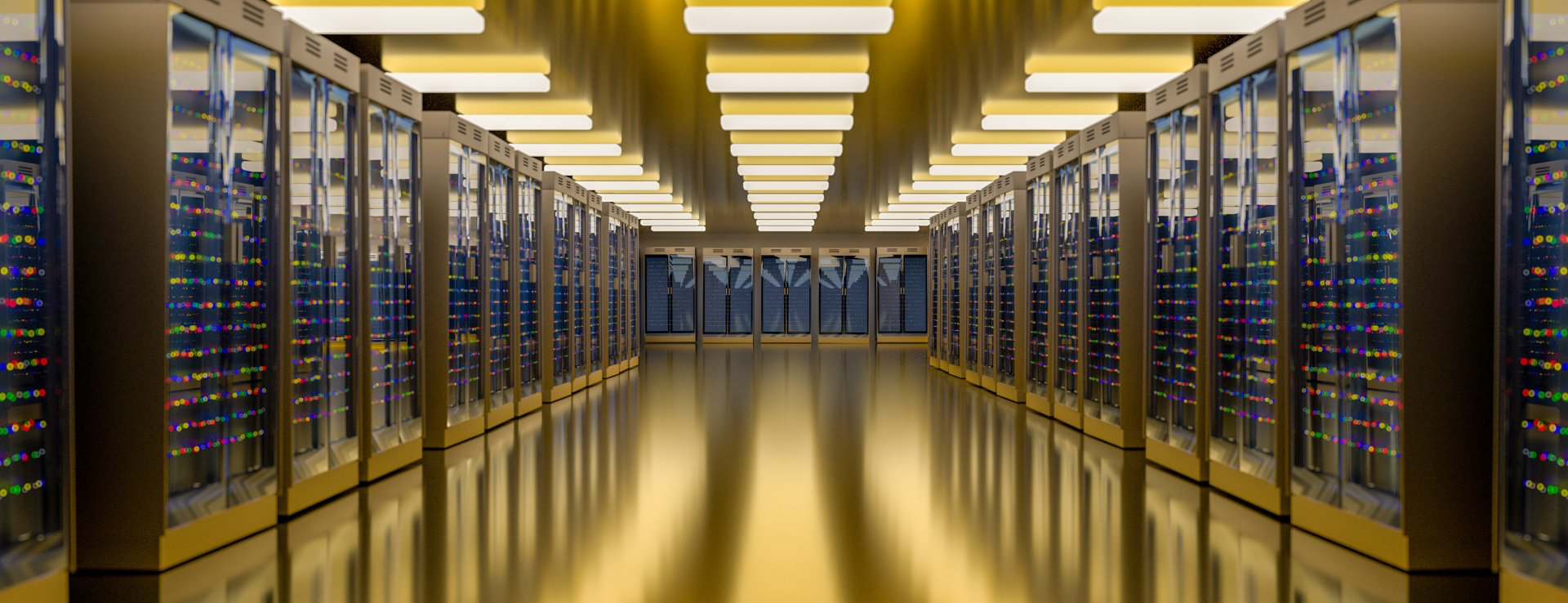
The cloud computing industry is at an important environmental inflection point. A single cloud computing data center can consume as much power as 50,000 homes. And all that power adds up to serious carbon costs. The carbon footprint of cloud computing is now larger than the airline industry’s. Considering other unintended consequences, like energy consumption, 50 million metric tons of hardware waste annually, and even acoustic waste, cloud computing has a big impact on the environment.
Part of the reason the cloud computing industry has become so environmentally expensive is because of how it grew. Once upon a time, real estate, electric power, and server equipment were all plentiful and cheap. So, when a large computing client expected an uptick in demand, the cloud provider would simply build more server farms, provision more equipment, and meet demand with a surplus of availability.
That approach, however, isn’t very efficient. Each server increases cooling demands, and each new computing center creates a large environmental footprint. To add insult to injury, most of the computing equipment experiences less than 50% utilization.
But times have changed. Server equipment is harder to find due to the global chip shortage. And environmental, social, and governance issues have become a greater priority for both cloud providers and consumers.
One cloud computing provider turned to Redpoint AI to help them create a more efficient computing environment.
They needed a solution that would:
- Reduce a cloud data center’s size, weight, and power (SWaP) demands. Excess computing hardware unnecessarily consumes power and cooling resources.
- Monitor and plan resource allocation. Client applications need contiguous space, running in a virtual machine on a single piece of hardware. To provide the best quality of service, they need to run on the best available hardware.
- Dynamically adapt to fluctuations in client resource requests. Rapidly changing resource requests historically meant adding new hardware to adapt to fluctuations in demand.
Redpoint AI used supervised and unsupervised machine learning to recognize and categorize the usage requirements of client applications. We found it was possible to identify which CPUs are used when and how much, so that the algorithm can regulate applications that are handed to the cloud provider. As a result, the cloud computing provider can:
- Provide the same or better quality of service with less hardware. The algorithm plays a high-tech Tetris game that allows client applications to fit better in the available space. That minimizes the hardware requirements and allowed the cloud provider to eliminate underutilized hardware.
- Allocate resource demands to the best available hardware. The algorithm enables real-time resource monitoring and regulation, even with non-linear parameters.
- Perform dynamic replanning. The algorithm enables dynamic replanning and migration, letting the cloud provider move virtual machines from one server to the next without impacting quality of service.
Increases in demand used to mean adding more hardware. By using the algorithm, the cloud provider can adapt dynamically, and in real time, to ever-fluctuating client demands. The algorithms help to efficiently use all of the available server space without impacting quality of service.
“There was a lot of wasted real estate on servers because they’re not being used to their full potential,” says Redpoint AI’s CEO Jeff Clark, PhD. “And algorithms like these are a great way to get the most out of your computing environment.”
As a result, the cloud provider has taken a step in the right direction from an environmental perspective. And lowered their operational costs in the process.
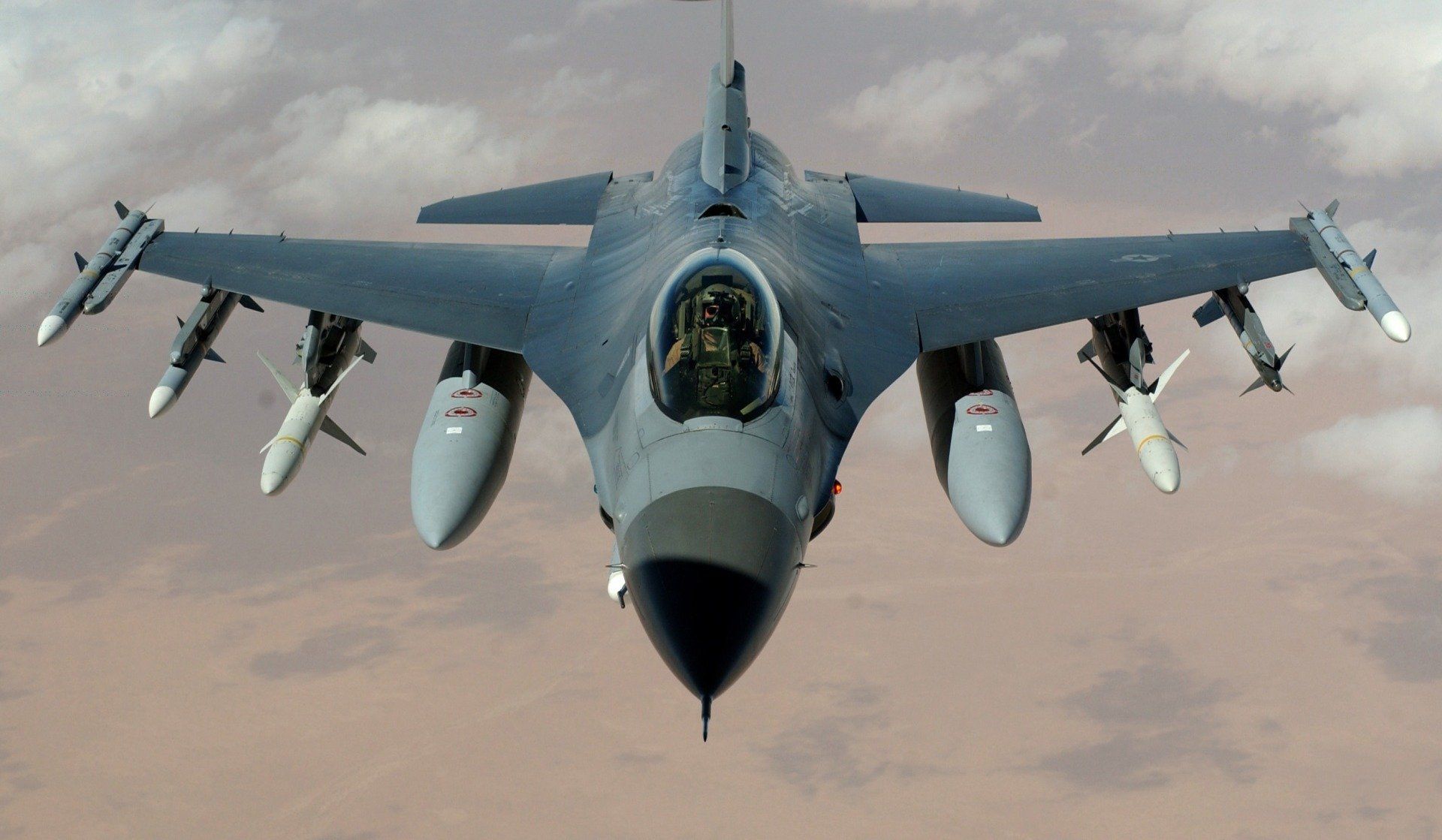
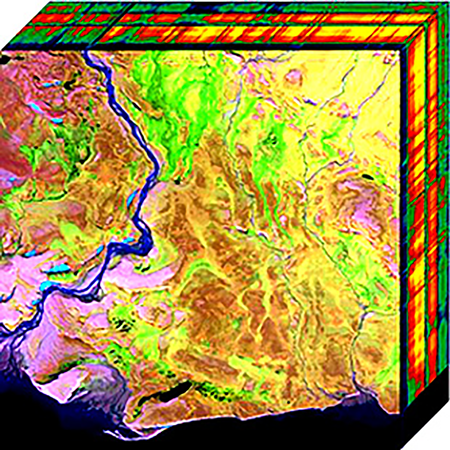