How ML is tackling the global silicon chip shortage
Applying ML to the silicon ingot manufacturing process reduces errors by more than 60%. And significantly increases production capacity for silicon chip manufacturers.
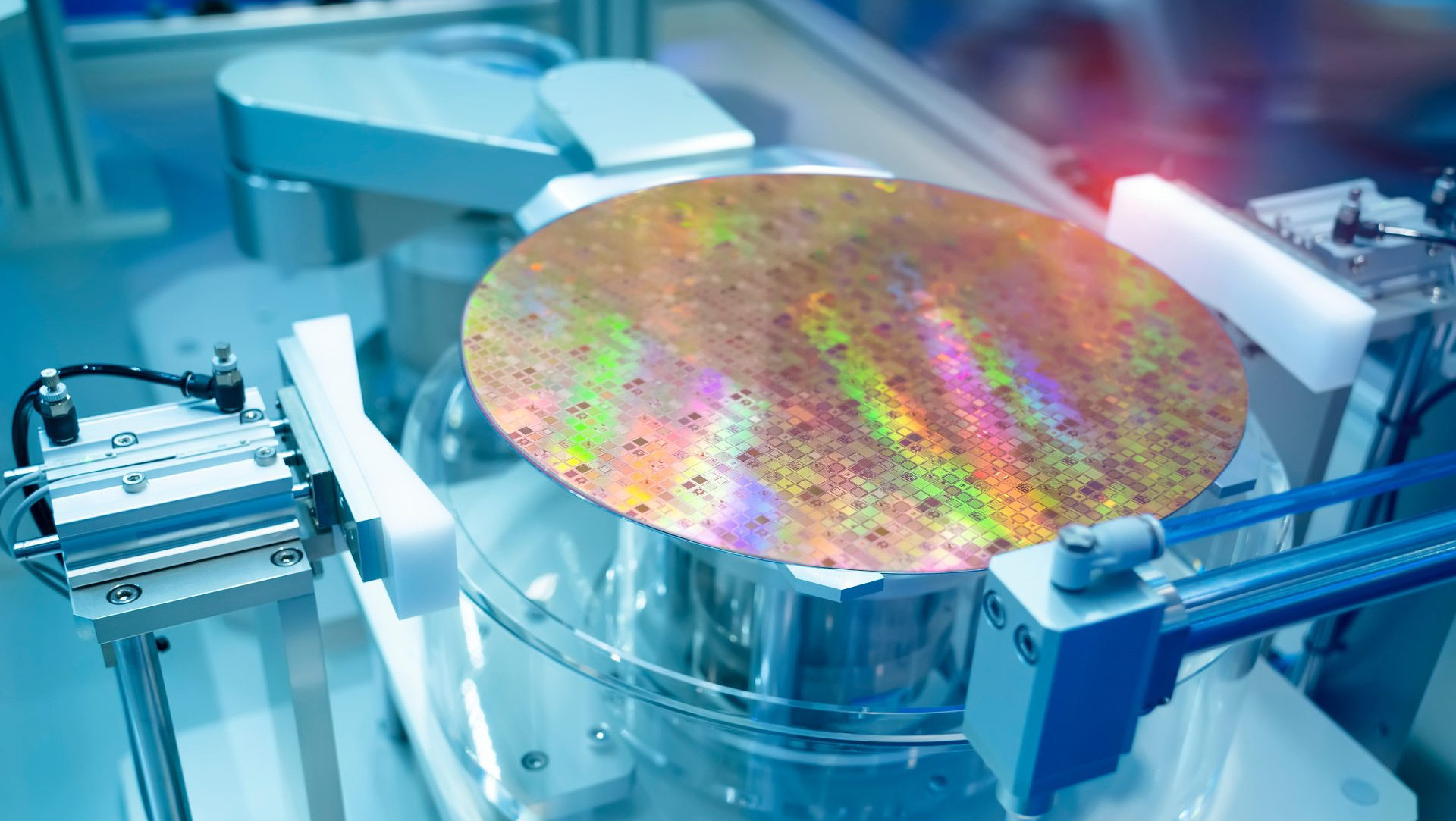
Silicon chips are found in an ever-increasing number of industrial and consumer goods. And the world is in the midst of a silicon chip shortage. One cause of the chip shortage is the manufacturing process. Making a silicon ingot – the raw material from which chips are cut – can take days, even weeks.
As a result, everything from laptops to cars are harder to find.
Making silicon ingots is a practiced, precise trade. Operators train for years to be able to identify the precise conditions that create a usable silicon ingot. Across the globe, chip foundries heat silicon to its melting point – a blistering 2570 degrees Fahrenheit. Then, the operator carefully watches the nearly invisible melted silicon to decide the exact moment to start pulling the seed (the starting point of an ingot) from the melted silicon.
Under perfect conditions, pulling the seed from the melted silicon produces what’s called the “neck,” a narrow tube that’s eventually widened to form the “head,” “shoulders,” and then the “body” of the ingot. Under ideal circumstances, the ingot’s perfect crystalline structure can then grow to a length of as many as eight feet. After the ingot is formed, it can be harvested and cut to create silicon wafers, chips, and microelectronics.
These wafers and chips become the brains of computers, smartphones, cars, and all sorts of consumer, industrial, government, and military computers.
However, the process is fraught with potential pitfalls. The conditions must be exactly right or the crystalline structure will fail to form correctly. Issues in the crystalline structure commonly start early in the process when the neck is formed. As a skilled operator watches the ingot form, they look for node lines – indicators that the crystal structure is good. If node lines fail to appear – or disappear – the process must be restarted. Sometimes, that’s after a few hours of work. Sometimes, and more devastatingly, that can be a day or two after a good ingot suddenly turned bad.
Each restart costs valuable time and resources. And making a single, good silicon ingot can take as many as three or more restarts – many days of trial, remelting, and restarting.
One silicon chip manufacturer turned to Redpoint AI for help.
They needed a solution that would:
- Reduce the number of issues in the silicon ingot manufacturing process. The process for manufacturing a silicon ingot has, historically, not been an exact science. It takes trial-and-error to make a good ingot.
- Help operators create a silicon ingot with a good crystalline structure. Silicon ingot manufacturers (operators), like most tradespeople, apprentice for years before become a master of their trade. Providing them with better tools would make it easier for them to make high quality ingots.
- Make a usable ingot in fewer attempts. Making a single good silicon ingot takes an average of three attempts. Since a single attempt takes as many as three or more days, failures mean significant losses in production capacity.
To solve these problems, Redpoint AI started a two-stage investigation. First by analyzing an archive of structured and unstructured data, then by applying machine learning to isolate the conditions that create a good crystalline structure.
With Redpoint AI, the silicon chip manufacturer was able to:
- Identify the parameters that create usable silicon ingots. After analyzing the data using machine learning, Redpoint AI was able to isolate which parameters were essential to creating a good ingot. Creating a good neck, for example, predicates a usable ingot. We identified which parameters lead to a strong crystalline structure throughout the entire process – from seed to tail.
- Provide operators with a precise set of conditions that create good crystalline structures. Redpoint AI’s algorithms can guide silicon operators through the most crucial points of the ingot manufacturing process.
- Make a usable ingot in just one attempt. After implementing Redpoint AI’s algorithms, the manufacturer can make successful ingots in fewer attempts – often just one.
Before Redpoint AI’s algorithms, the silicon ingot manufacturer was using a trial-and-error process to create their ingots. The process relied on expert tradespeople to be successful in 33% of attempts.
“We used structured and unstructured FMV data to increase their success rate,” says Redpoint AI CEO Jeff Clark, PhD. “The process was very reliant on craft and good luck. But the operators can use our algorithm to increase their success rate to nearly 100%. For the silicon chip manufacturers, that results in a massive increase in capacity.”
Now, the silicon operator can reduce the time to create a usable ingot by days (not hours), making a significant advance in overcoming the worldwide chip shortage.
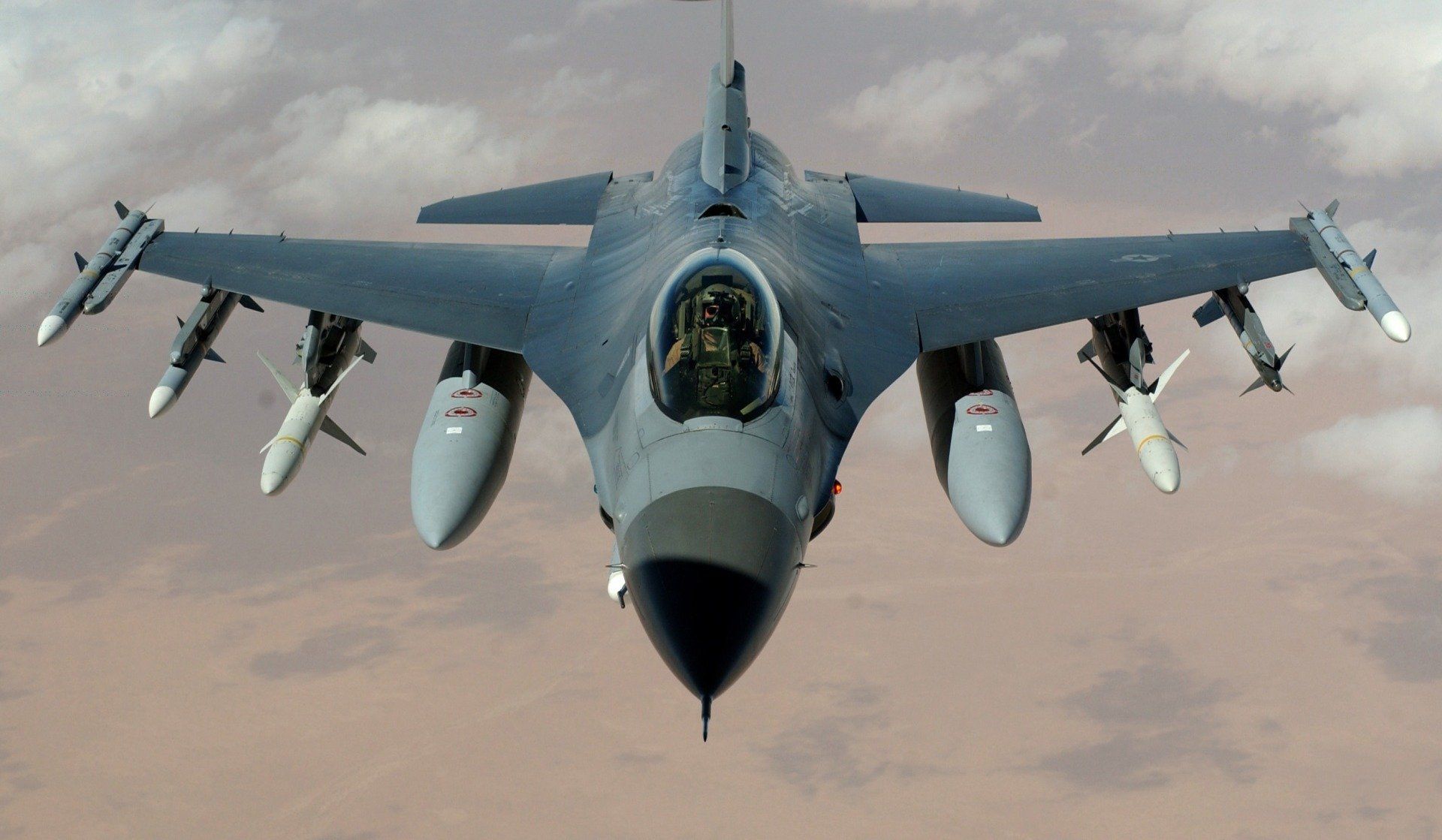
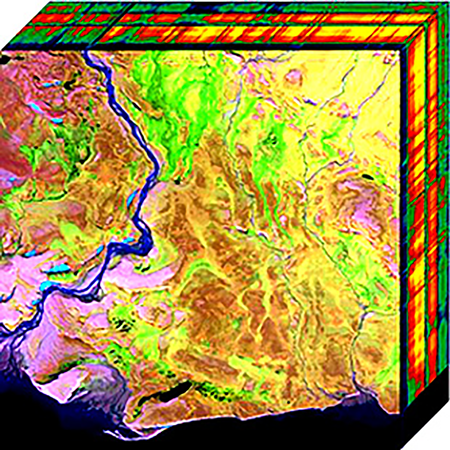