10 Ways SNNs Mimic the Architectural Wonders of the Brain
Machine learning that draws inspiration from the brain's intricate structures and connectivity.
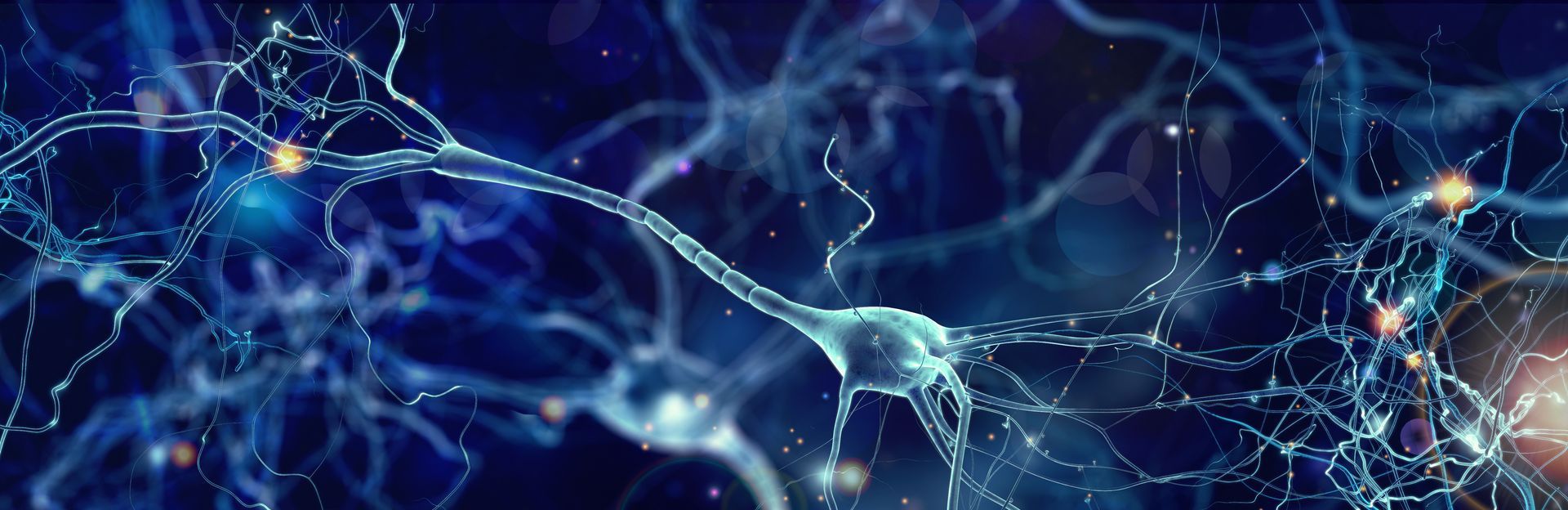
The human brain remains the pinnacle of biological computing - an intricate network of 86 billion neurons that gives rise to consciousness, intelligence, and the full breadth of human cognition. Yet while neuroscience has revealed many of the brain's secrets, we still lack the ability to recreate its astonishing capabilities in artificial systems.
Spiking neural networks (SNNs) offer a promising path to emulate the brain's biological complexity. By incorporating concepts like temporal dynamics, adaptation, and noise, SNNs aim to mirror the behavior of actual neurons down to the millisecond scale. While a perfect replica remains elusive, these brain-inspired networks are bringing us closer to natural intelligence.
In this article, we'll explore some of the key techniques researchers are applying to make SNNs more biologically accurate, from mimicking neural architecture to replicating molecular dynamics. These approaches attempt to reverse engineer the brain's extraordinary abilities and implement them in silicon circuits and computer models. As the line between artificial and biological blurs, SNNs represent an exciting frontier in building truly brain-like intelligence.
- Biologically-Inspired Models: One way to make SNNs resemble biological neurons more closely is to derive models based on actual physiological observations. The Hodgkin-Huxley model and the Izhikevich model are examples that describe the spiking behavior of neurons with a higher degree of biological realism than simpler models.
- Synaptic Plasticity: The adaptation of synapses is at the heart of learning and memory in biological systems. Incorporating spike-timing-dependent plasticity (STDP) into SNNs, where the strength of synapses changes based on the timing of spikes, can make these networks behave more like biological neurons.
- Neurotransmitters and Neuromodulation: In the brain, various neurotransmitters (like dopamine, serotonin, etc.) modulate neural activity. Incorporating different types of neurotransmitter dynamics and neuromodulation into SNNs might help in capturing some of the richness of biological neural networks.
- Network Architecture: The architecture of the brain's neural network, with its layers, hierarchies, and recurrent connections, plays a critical role in its function. Mimicking these structures, like cortical columns and brain regions with specific functions, might lead to more biologically accurate models.
- Incorporate Noise: Biological systems operate in a noisy environment, and this noise is believed to play a vital role in their computation. Introducing noise to SNNs, such that they can operate robustly in its presence, can potentially make them more biologically accurate.
- Energy Efficiency: One of the marvels of the human brain is its energy efficiency. Designing SNNs to be energy efficient, especially when implemented on neuromorphic hardware, could be another step towards mimicking biological precision.
- Multimodal Integration: The human brain processes information from a variety of sources (vision, sound, touch, etc.) in an integrated manner. Building SNNs that can take multiple types of input and integrate this information could help in replicating some aspects of biological computation.
- Adaptive Thresholding: In biological neurons, the threshold for firing can change based on the recent activity of the neuron. This adaptive threshold mechanism can be incorporated into SNNs to make them more dynamic and closer to their biological counterparts.
- Homeostasis: Neurons have mechanisms to maintain certain activity levels over time, adapting their responsiveness based on external inputs. Incorporating similar mechanisms into SNNs can provide more stability and biological resemblance.
- Molecular Dynamics and Processes: At an even deeper level, understanding and replicating the molecular dynamics within neurons, such as ion channel functions, could provide insights into the precise operations of biological systems.
Achieving a complete mimicry of biological precision is a vast challenge, given the complexity of biological systems. However, as we continue to refine our models and approaches, and as we gain deeper insights into how biological systems operate, the gap between artificial and biological systems will likely narrow.
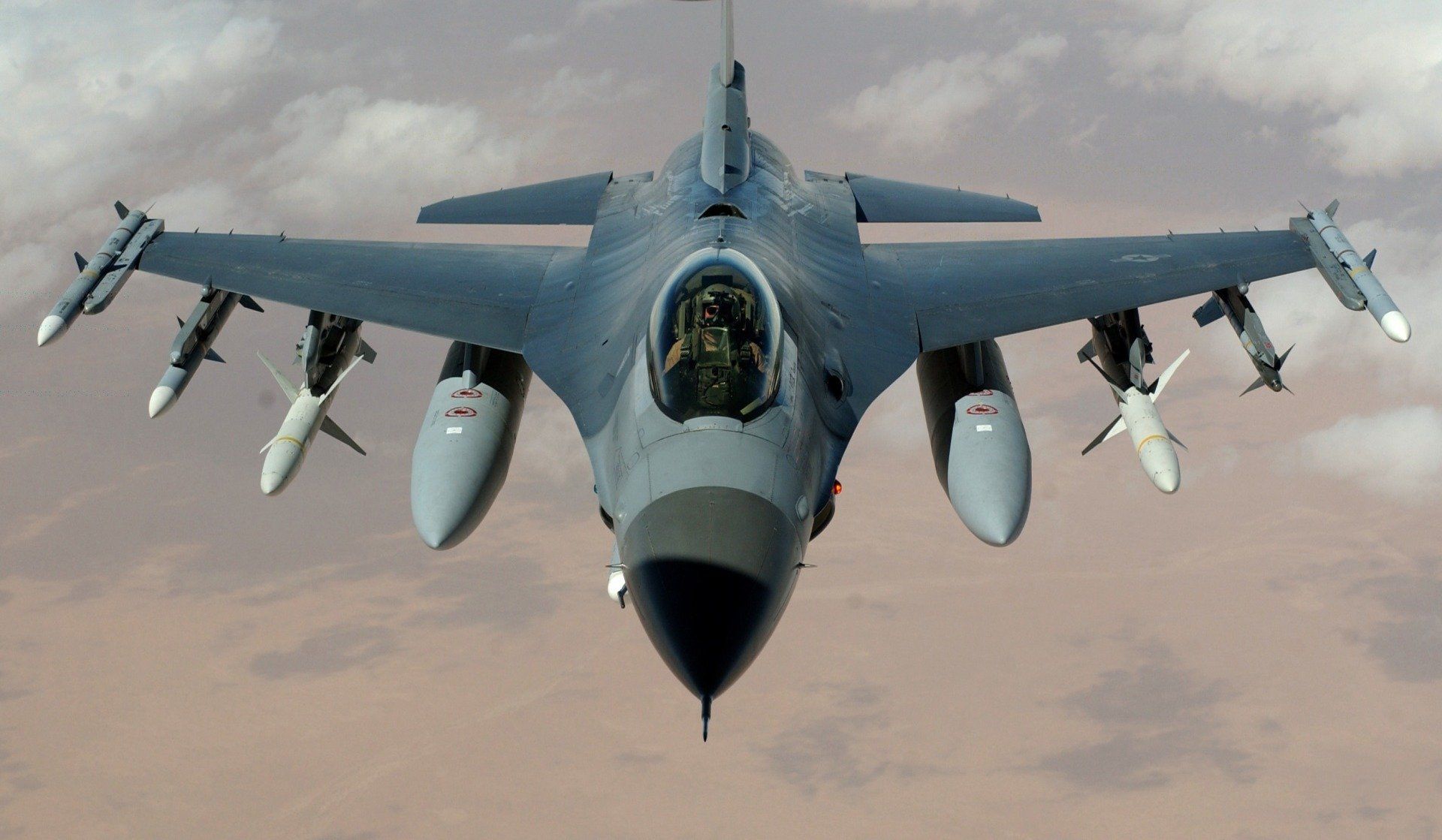
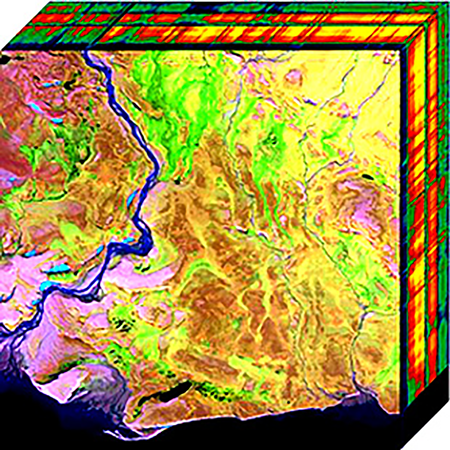